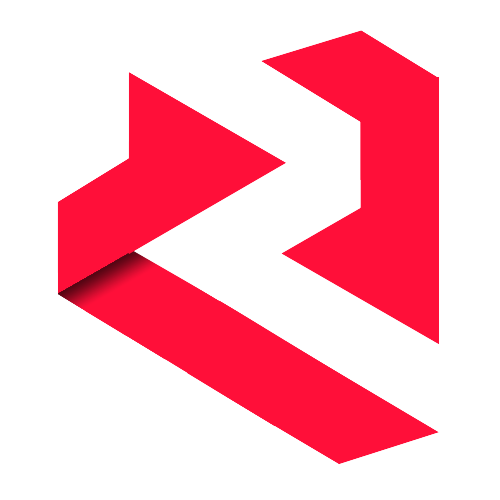
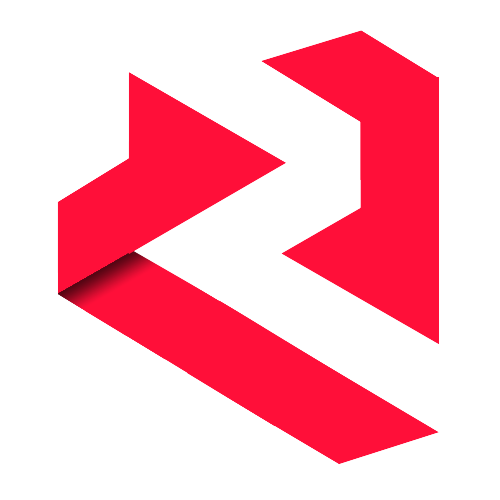
Every year, millions of Muslims worldwide come to Mecca to perform the Hajj.
In order to maintain the security of the pilgrims, the Saudi government has installed about 5000
closed circuit television (CCTV) cameras to monitor crowd activity efficiently.
In this case, Our Abnormal Human Activity Recognition can make a change in surveillance technology
which can be monitored human activites efficiently and effectively with less human interventions.
The existing method is incapable of extracting discriminative features from surveillance videos as pre-trained weights of different architectures were used. As the first step of the proposed framework, a lightweight CNN model is trained on our own pilgrim's dataset to detect pilgrims from the surveillance cameras. These preprocessed salient frames are passed to a lightweight CNN model for spatial features extraction in the second step. In the third step, a Long Short Term Memory network (LSTM) is developed to extract temporal features. Finally, in the last step, in the case of violent activity or accidents, the proposed system will generate an alarm in real time to inform law enforcement agencies to take appropriate action, thus helping to avoid accidents and stampedes.